This digital transformation era underscores the paramount role of data in propelling research and development (R&D) forward. In fact, its significance has never been more pronounced.
Yet, this exponential surge in data utilization brings to light many consequential issues related to privacy and security which can’t be ignored.
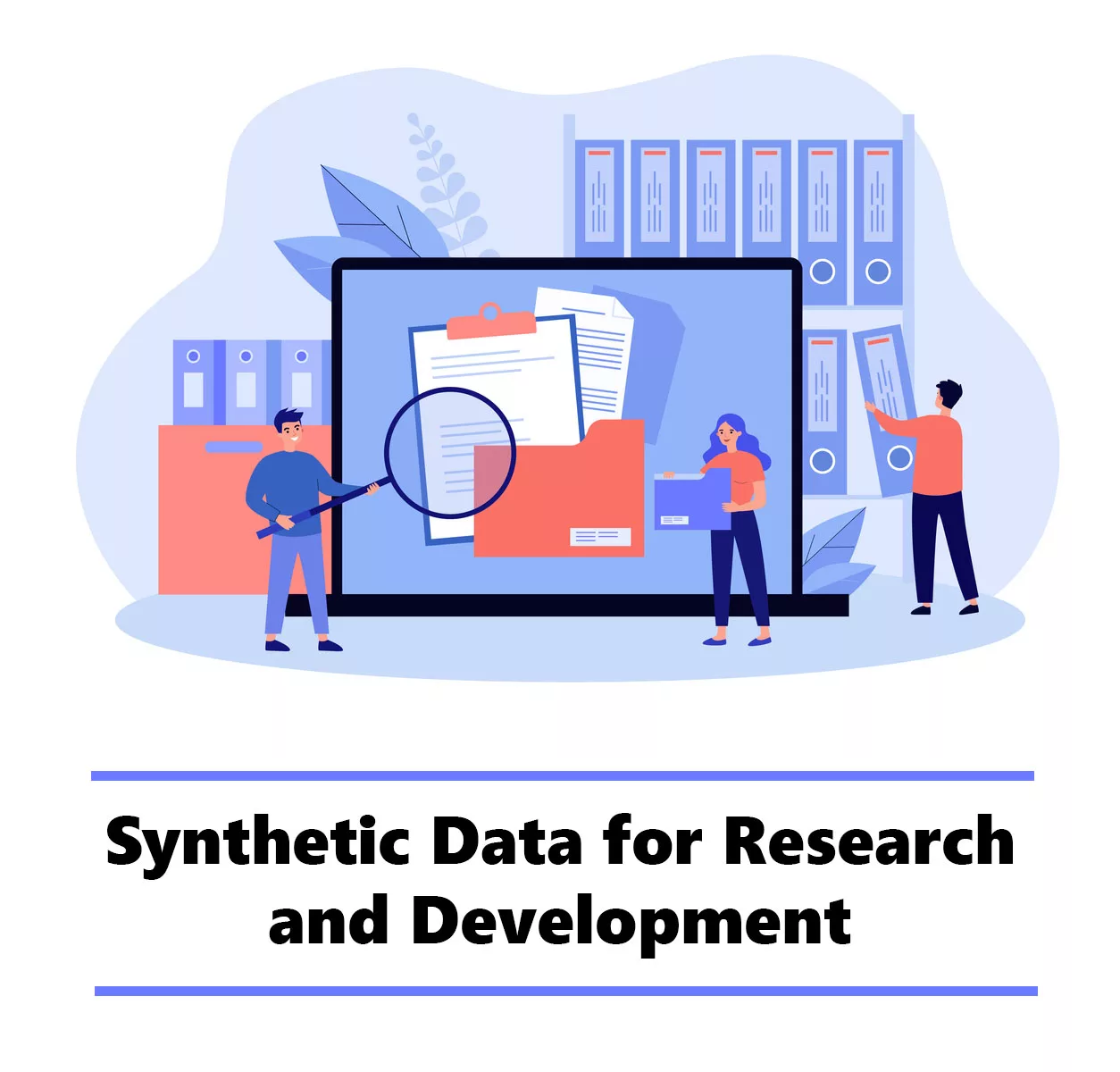
Conventional methods for collecting data frequently necessitate the handling of sensitive information-a practice which spawns ethical quandaries as well as regulatory obstacles.
Here, synthetic data introduces a groundbreaking solution. It not only mitigates these concerns but also propels innovation in R&D towards unexplored possibilities.
The Problem: Classic Data Gathering
Collecting real-life data for a research study is fraught with various challenges, more specifically in the privacy and safety domain. As the frequency of high-profile data breaches increases, the public and regulatory bodies constantly heighten their demands for adequate measures that ensure compliant data protection.
As sensitive information is involved in research, researchers have to make their way through a complicated maze of legal factors they must take into account which prevents the development of essential studies.
Additionally, stricter privacy regulations and people’s reluctance to provide their personally identifiable information limit data access of certain types – for instance, medical records or financial transactions. Research scope and depth are limited because of a shortage of such data, thus delaying the progress in areas such as healthcare, finance, etc.
The Solution: What is Synthetic Data?
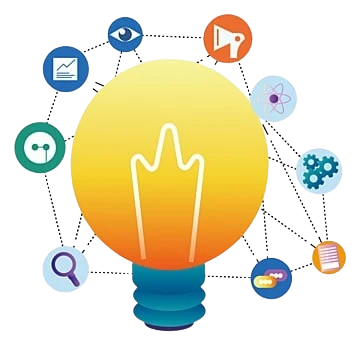
Synthetic data presents a groundbreaking solution to privacy-related challenges with its ability to mimic the statistical characteristics of real data while excluding identifiable information. Indeed, it revolutionizes research by offering an avenue for conducting profound investigations with robust data privacy management.
Privacy Preservation: The main advantage of synthetic data is privacy, as researchers manipulate very detailed and diverse datasets — but without compromising confidentiality by creating non-representative information about real individuals.
This approach does not only strengthen the integrity of sensitive data but also amplifies efficiency during research processes- avoiding comprehensive ethical considerations and rigorous compliance with standard data protection guidelines.
Overcoming Data Scarcity: By the use of synthetic data, researchers can deal with the problem of limited real data availability especially in situations where minimal real date is available.
For instance, privacy regulations often pose obstacles to medical research in getting patient records. Nevertheless, with the aid of synthetic data they are able to build realistic datasets that can be used for their experimentations and computational analysis.
Enhanced Collaboration and Data Sharing: The use of synthetic data contributes to the development of cooperation among researchers and facilitates the implementation of data sharing practice.
It resides in its non-sensitive nature, which makes it easy for cross institutional dissemination thus creating a milieu of collaboration that accelerates innovation fast.
Case Studies:
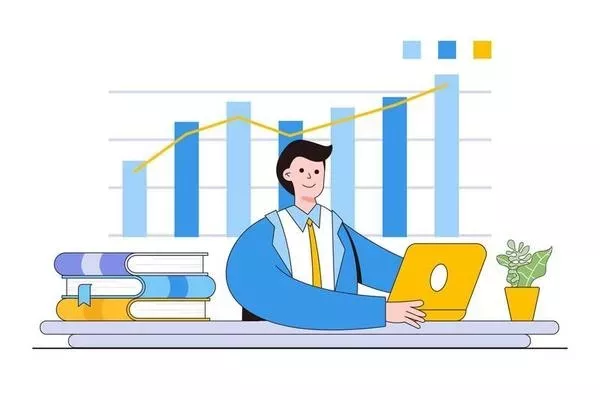
To illustrate the impact of synthetic data, let’s explore a couple of case studies:
Healthcare Research:
Researchers, in a study that zeroes in on comprehending the spread of infectious diseases, utilize synthetic data to simulate an array of scenarios- all without jeopardizing patient privacy.
This approach enables them to model distinct epidemiological patterns and consequently devise strategies for public health with enhanced efficacy.
Financial Modeling:
Constrained by stringent privacy regulations, financial institutions utilized synthetic data for building and testing predictive risk assessment models. This strategy enhanced their analysis accuracy; moreover, it fostered collaboration among diverse organizations in the industry.
Best Techniques
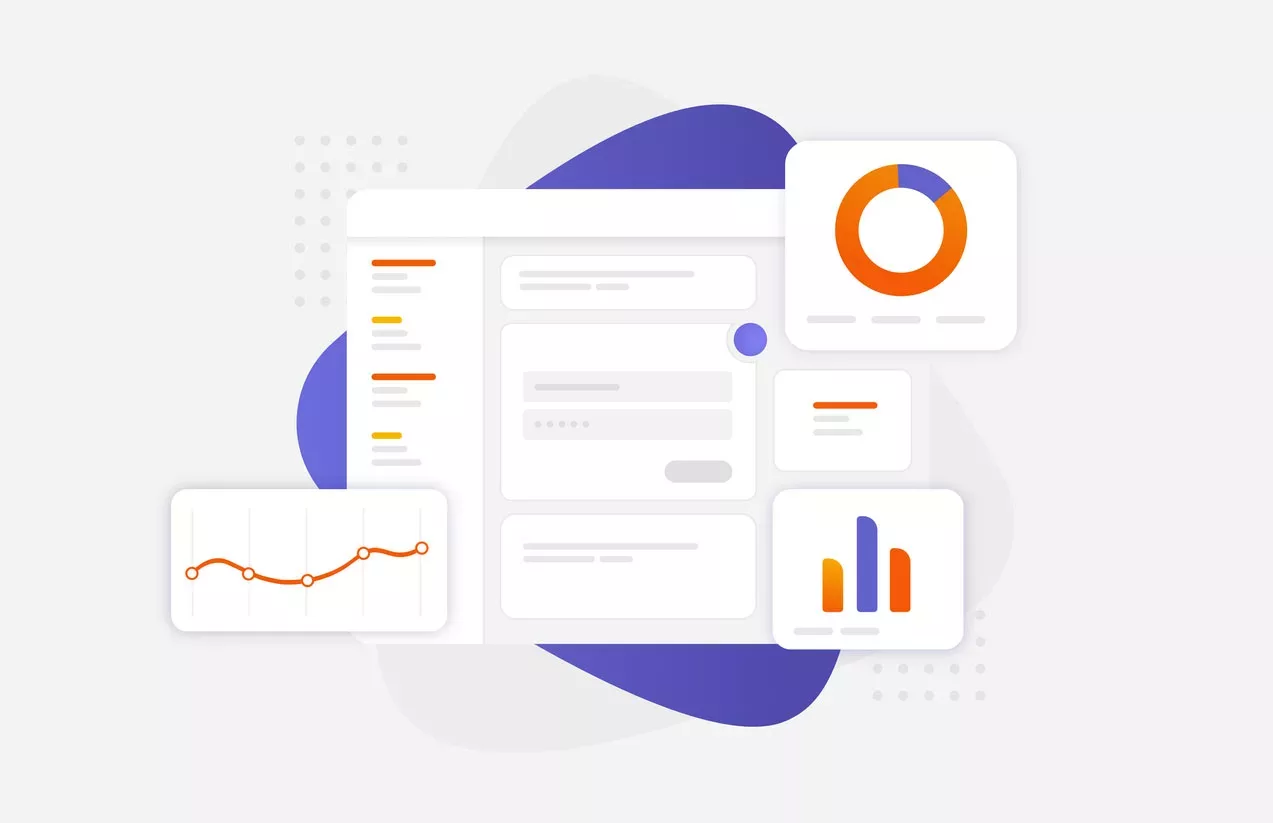
Sophisticated techniques that capture the statistical properties of real data —while safeguarding against identifiability issues— are necessary for generating high-quality synthetic data. The most effective methods used by synthetic data generation tools in this pursuit include:
Generative Adversarial Networks (GANs): Two neural networks, a generator and a discriminator, form the core of Generative Adversarial Networks (GANs) which have achieved extensive popularity for synthetic data generation.
These two components undergo simultaneous training: the generator fabricates synthetic data while the discriminator assesses its authenticity by distinguishing between real and artificial inputs. This adversarial training process results in the generator producing increasingly realistic synthetic data.
Copula-Based Modeling: Mathematical functions, known as copulas, depict the dependency structure between random variables. Copula-based models enable us to generate synthetic data while maintaining observed statistical dependencies in the original dataset. This method proves especially valuable when preserving correlation structures among variables emerges as a critical factor- as is often in financial modeling.
Variational Autoencoders (VAEs): Another neural network-based approach for generating synthetic data is the Variational Autoencoders (VAEs). These models learn the underlying structure of input data and subsequently produce new points- each bearing similar features. VAEs excel in capturing a dataset’s latent space, thereby enabling creation of diverse yet realistic synthetic samples.
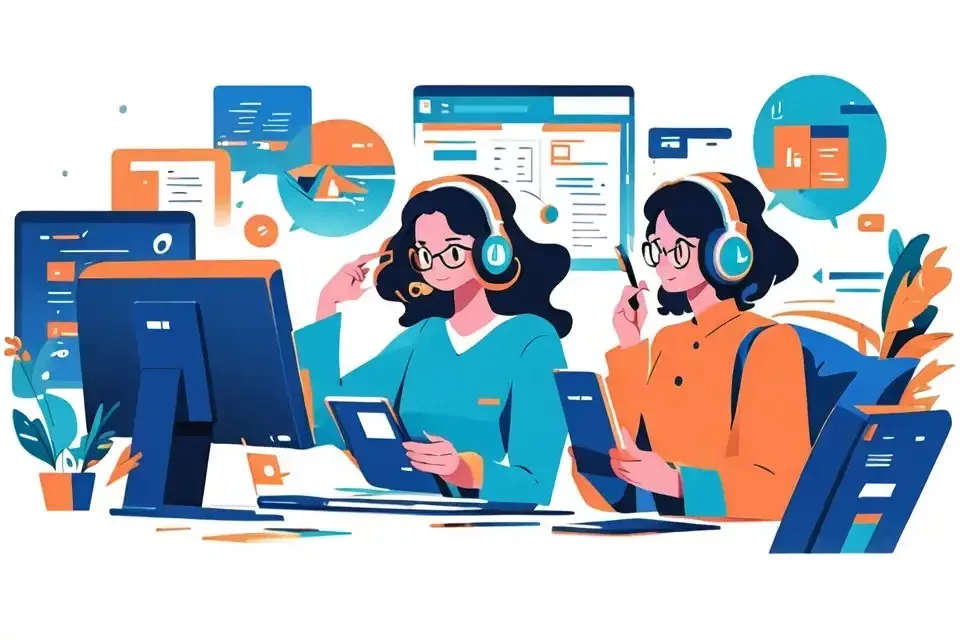
Markov Chain Monte Carlo (MCMC) Methods: By means of a simulated Markov chain, MCMC techniques produce new samples resembling the data distribution that is a characteristic feature of actual settings.
This approach is often used in cases when the sequential dependencies of data are very important for capturing, as this is usually can be seen financial time series or where one has to study epidemiology patterns.
Differential Privacy: The technique of differential privacy is especially applicable to sensitive fields such as healthcare and social sciences since it involves randomness with data allowing for fluctuations, thereby ensuring that individuals who are identified within datasets cannot be singled out.
In specific applications, where secrecy of personal information is even more paramount than utility, differential privacy ensures both; protection and usefulness by guaranteeing both statistical properties and unparalleled degrees of security for the data.
Data Masking and Perturbation: Data masking involves modifying or obscuring specific elements of the original data to create synthetic datasets. This can include techniques like randomization, noise injection, or shuffling.
Conclusions
Synthetic data possesses immense potential for unraveling research and development complexities: it enables the scholars to explore new frontiers in different fields. They become empowered through data democratization and overcoming privacy concerns and scarcity of data, a success brought about by blending synthetic data. This critical evolution leads towards the future where innovation is free from obstacles—the limitations that are part of classic data gathering methods.